The first GPT-4-class AI model anyone can download has arrived: Llama 405B
A new llama emerges —
“Open source AI is the path forward,” says Mark Zuckerberg, misusing the term.
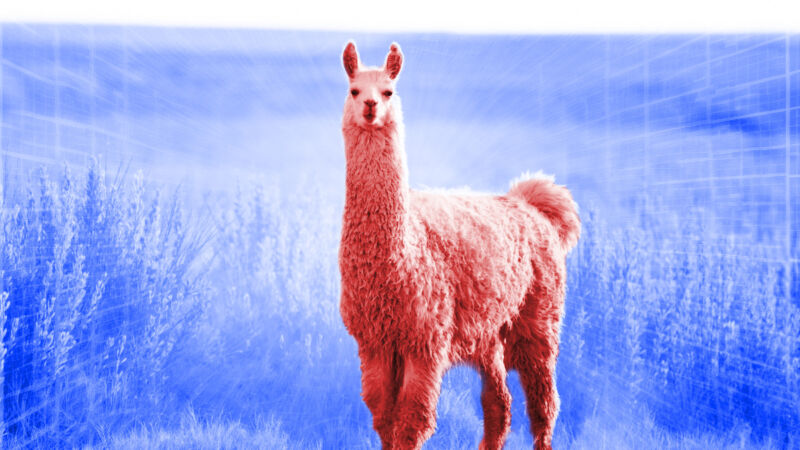
In the AI world, there’s a buzz in the air about a new AI language model released Tuesday by Meta: Llama 3.1 405B. The reason? It’s potentially the first time anyone can download a GPT-4-class large language model (LLM) for free and run it on their own hardware. You’ll still need some beefy hardware: Meta says it can run on a “single server node,” which isn’t desktop PC-grade equipment. But it’s a provocative shot across the bow of “closed” AI model vendors such as OpenAI and Anthropic.
“Llama 3.1 405B is the first openly available model that rivals the top AI models when it comes to state-of-the-art capabilities in general knowledge, steerability, math, tool use, and multilingual translation,” says Meta. Company CEO Mark Zuckerberg calls 405B “the first frontier-level open source AI model.”
In the AI industry, “frontier model” is a term for an AI system designed to push the boundaries of current capabilities. In this case, Meta is positioning 405B among the likes of the industry’s top AI models, such as OpenAI’s GPT-4o, Claude’s 3.5 Sonnet, and Google Gemini 1.5 Pro.
A chart published by Meta suggests that 405B gets very close to matching the performance of GPT-4 Turbo, GPT-4o, and Claude 3.5 Sonnet in benchmarks like MMLU (undergraduate level knowledge), GSM8K (grade school math), and HumanEval (coding).
But as we’ve noted many times since March, these benchmarks aren’t necessarily scientifically sound or translate to the subjective experience of interacting with AI language models. In fact, this traditional slate of AI benchmarks is so generally useless to laypeople that even Meta’s PR department now just posts a few images of charts and doesn’t even try to explain them in any detail.
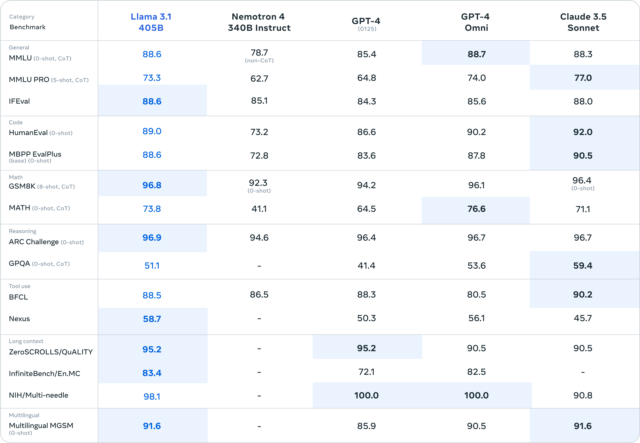
Enlarge / A Meta-provided chart that shows Llama 3.1 405B benchmark results versus other major AI models.
We’ve instead found that measuring the subjective experience of using a conversational AI model (through what might be called “vibemarking”) on A/B leaderboards like Chatbot Arena is a better way to judge new LLMs. In the absence of Chatbot Arena data, Meta has provided the results of its own human evaluations of 405B’s outputs that seem to show Meta’s new model holding its own against GPT-4 Turbo and Claude 3.5 Sonnet.
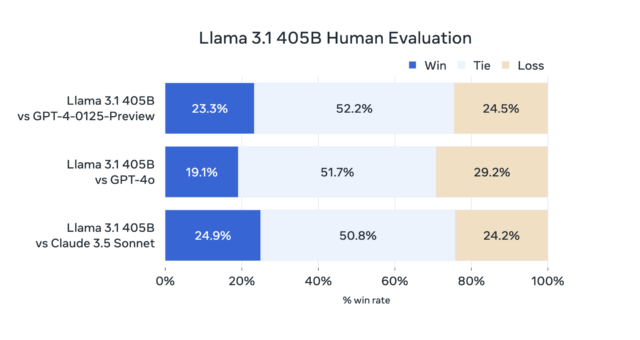
Enlarge / A Meta-provided chart that shows how humans rated Llama 3.1 405B’s outputs compared to GPT-4 Turbo, GPT-4o, and Claude 3.5 Sonnet in its own studies.
Whatever the benchmarks, early word on the street (after the model leaked on 4chan yesterday) seems to match the claim that 405B is roughly equivalent to GPT-4. It took a lot of expensive computer training time to get there—and money, of which the social media giant has plenty to burn. Meta trained the 405B model on over 15 trillion tokens of training data scraped from the web (then parsed, filtered, and annotated by Llama 2), using more than 16,000 H100 GPUs.
So what’s with the 405B name? In this case, “405B” means 405 billion parameters, and parameters are numerical values that store trained information in a neural network. More parameters translate to a larger neural network powering the AI model, which generally (but not always) means more capability, such as better ability to make contextual connections between concepts. But larger-parameter models have a tradeoff in needing more computing power (AKA “compute”) to run.
We’ve been expecting the release of a 400 billion-plus parameter model of the Llama 3 family since Meta gave word that it was training one in April, and today’s announcement isn’t just about the biggest member of the Llama 3 family: There’s an entirely new iteration of improved Llama models with the designation “Llama 3.1.” That includes upgraded versions of its smaller 8B and 70B models, which now feature multilingual support and an extended context length of 128,000 tokens (the “context length” is roughly the working memory capacity of the model, and “tokens” are chunks of data used by LLMs to process information).
Meta says that 405B is useful for long-form text summarization, multilingual conversational agents, and coding assistants and for creating synthetic data used to train future AI language models. Notably, that last use-case—allowing developers to use outputs from Llama models to improve other AI models—is now officially supported by Meta’s Llama 3.1 license for the first time.
Abusing the term “open source”
Llama 3.1 405B is an open-weights model, which means anyone can download the trained neural network files and run them or fine-tune them. That directly challenges a business model where companies like OpenAI keep the weights to themselves and instead monetize the model through subscription wrappers like ChatGPT or charge for access by the token through an API.
Fighting the “closed” AI model is a big deal to Mark Zuckerberg, who simultaneously released a 2,300-word manifesto today on why the company believes in open releases of AI models, titled, “Open Source AI Is the Path Forward.” More on the terminology in a minute. But briefly, he writes about the need for customizable AI models that offer user control and encourage better data security, higher cost-efficiency, and better future-proofing, as opposed to vendor-locked solutions.
All that sounds reasonable, but undermining your competitors using a model subsidized by a social media war chest is also an efficient way to play spoiler in a market where you might not always win with the most cutting-edge tech. That benefits Meta, Zuckerberg says, because he doesn’t want to get locked into a system where companies like his have to pay a toll to access AI capabilities, drawing comparisons to “taxes” Apple levies on developers through its App Store.
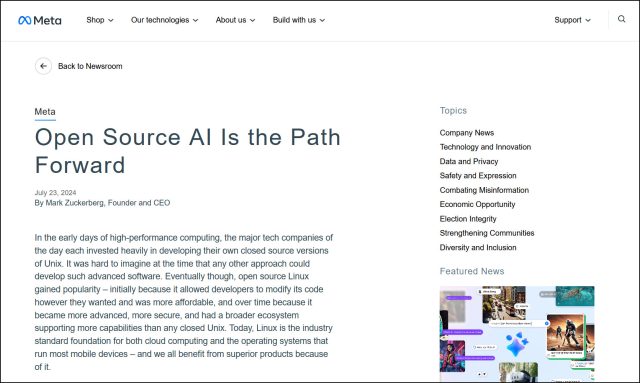
Enlarge / A screenshot of Mark Zuckerberg’s essay, “Open Source AI Is the Path Forward,” published on July 23, 2024.
So, about that “open source” term. As we first wrote in an update to our Llama 2 launch article a year ago, “open source” has a very particular meaning that has traditionally been defined by the Open Source Initiative. The AI industry has not yet settled on terminology for AI model releases that ship either code or weights with restrictions (such as Llama 3.1) or that ship without providing training data. We’ve been calling these releases “open weights” instead.
Unfortunately for terminology sticklers, Zuckerberg has now baked the erroneous “open source” label into the title of his potentially historic aforementioned essay on open AI releases, so fighting for the correct term in AI may be a losing battle. Still, his usage annoys people like independent AI researcher Simon Willison, who likes Zuckerberg’s essay otherwise.
“I see Zuck’s prominent misuse of ‘open source’ as a small-scale act of cultural vandalism,” Willison told Ars Technica. “Open source should have an agreed meaning. Abusing the term weakens that meaning which makes the term less generally useful, because if someone says ‘it’s open source,’ that no longer tells me anything useful. I have to then dig in and figure out what they’re actually talking about.”
The Llama 3.1 models are available for download through Meta’s own website and on Hugging Face. They both require providing contact information and agreeing to a license and an acceptable use policy, which means that Meta can technically legally pull the rug out from under your use of Llama 3.1 or its outputs at any time.
The first GPT-4-class AI model anyone can download has arrived: Llama 405B Read More »