EmTech Digital 2024: A thoughtful look at AI’s pros and cons with minimal hype
Massachusetts Institute of Sobriety —
At MIT conference, experts explore AI’s potential for “human flourishing” and the need for regulation.
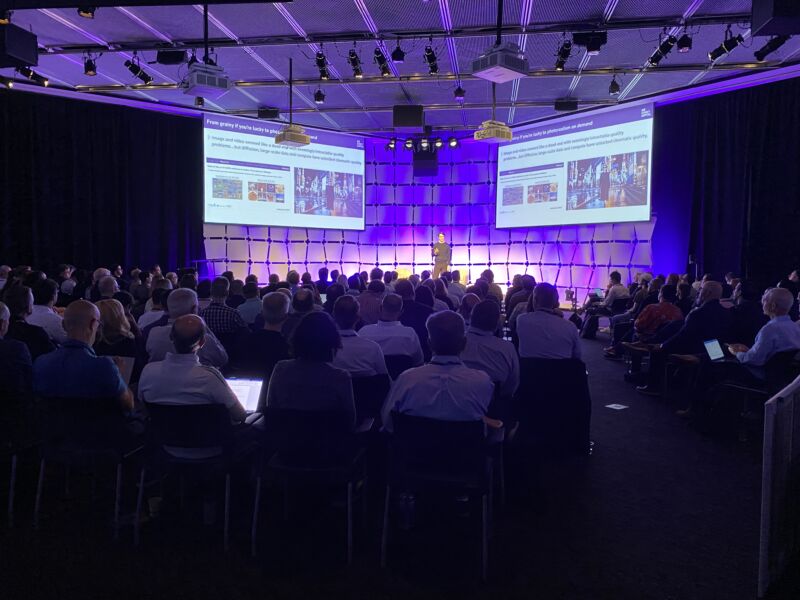
Enlarge / Nathan Benaich of Air Street Capital delivers the opening presentation on the state of AI at EmTech Digital 2024 on May 22, 2024.
Benj Edwards
CAMBRIDGE, Massachusetts—On Wednesday, AI enthusiasts and experts gathered to hear a series of presentations about the state of AI at EmTech Digital 2024 on the Massachusetts Institute of Technology’s campus. The event was hosted by the publication MIT Technology Review. The overall consensus is that generative AI is still in its very early stages—with policy, regulations, and social norms still being established—and its growth is likely to continue into the future.
I was there to check the event out. MIT is the birthplace of many tech innovations—including the first action-oriented computer video game—among others, so it felt fitting to hear talks about the latest tech craze in the same building that hosts MIT’s Media Lab on its sprawling and lush campus.
EmTech’s speakers included AI researchers, policy experts, critics, and company spokespeople. A corporate feel pervaded the event due to strategic sponsorships, but it was handled in a low-key way that matches the level-headed tech coverage coming out of MIT Technology Review. After each presentation, MIT Technology Review staff—such as Editor-in-Chief Mat Honan and Senior Reporter Melissa Heikkilä—did a brief sit-down interview with the speaker, pushing back on some points and emphasizing others. Then the speaker took a few audience questions if time allowed.
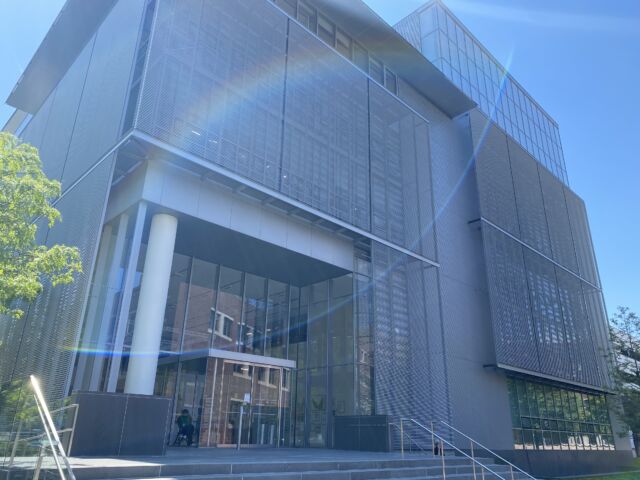
Enlarge / EmTech Digital 2024 took place in building E14 on MIT’s Campus in Cambridge, MA.
Benj Edwards
The conference kicked off with an overview of the state of AI by Nathan Benaich, founder and general partner of Air Street Capital, who rounded up news headlines about AI and several times expressed a favorable view toward defense spending on AI, making a few people visibly shift in their seats. Next up, Asu Ozdaglar, deputy dean of Academics at MIT’s Schwarzman College of Computing, spoke about the potential for “human flourishing” through AI-human symbiosis and the importance of AI regulation.
Kari Ann Briski, VP of AI Models, Software, and Services at Nvidia, highlighted the exponential growth of AI model complexity. She shared a prediction from consulting firm Gartner research that by 2026, 50 percent of customer service organizations will have customer-facing AI agents. Of course, Nvidia’s job is to drive demand for its chips, so in her presentation, Briski painted the AI space as an unqualified rosy situation, assuming that all LLMs are (and will be) useful and reliable, despite what we know about their tendencies to make things up.
The conference also addressed the legal and policy aspects of AI. Christabel Randolph from the Center for AI and Digital Policy—an organization that spearheaded a complaint about ChatGPT to the FTC last year—gave a compelling presentation about the need for AI systems to be human-centered and aligned, warning about the potential for anthropomorphic models to manipulate human behavior. She emphasized the importance of demanding accountability from those designing and deploying AI systems.
-
Asu Ozdaglar, deputy dean of Academics at MIT’s Schwarzman College of Computing, spoke about the potential for “human flourishing” through AI-human symbiosis at EmTech Digital on May 22, 2024.
Benj Edwards
-
Asu Ozdaglar, deputy dean of Academics at MIT’s Schwarzman College of Computing spoke with MIT Technology Review Editor-in-Chief Mat Honan at EmTech Digital on May 22, 2024.
Benj Edwards
-
Kari Ann Briski, VP of AI Models, Software, and Services at NVIDIA, highlighted the exponential growth of AI model complexity at EmTech Digital on May 22, 2024.
Benj Edwards
-
MIT Technology Review Senior Reporter Melissa Heikkilä introduces a speaker at EmTech Digital on May 22, 2024.
Benj Edwards
-
After her presentation, Christabel Randolph from the Center for AI and Digital Policy sat with MIT Technology Review Senior Reporter Melissa Heikkilä at EmTech Digital on May 22, 2024.
Benj Edwards
-
Lawyer Amir Ghavi provided an overview of the current legal landscape surrounding AI at EmTech Digital on May 22, 2024.
Benj Edwards
-
Lawyer Amir Ghavi provided an overview of the current legal landscape surrounding AI at EmTech Digital on May 22, 2024.
Benj Edwards
Amir Ghavi, an AI, Tech, Transactions, and IP partner at Fried Frank LLP, who has defended AI companies like Stability AI in court, provided an overview of the current legal landscape surrounding AI, noting that there have been 24 lawsuits related to AI so far in 2024. He predicted that IP lawsuits would eventually diminish, and he claimed that legal scholars believe that using training data constitutes fair use. He also talked about legal precedents with photocopiers and VCRs, which were both technologies demonized by IP holders until courts decided they constituted fair use. He pointed out that the entertainment industry’s loss on the VCR case ended up benefiting it by opening up the VHS and DVD markets, providing a brand new revenue channel that was valuable to those same companies.
In one of the higher-profile discussions, Meta President of Global Affairs Nick Clegg sat down with MIT Technology Review Executive Editor Amy Nordrum to discuss the role of social media in elections and the spread of misinformation, arguing that research suggests social media’s influence on elections is not as significant as many believe. He acknowledged the “whack-a-mole” nature of banning extremist groups on Facebook and emphasized the changes Meta has undergone since 2016, increasing fact-checkers and removing bad actors.
EmTech Digital 2024: A thoughtful look at AI’s pros and cons with minimal hype Read More »