Quantum computing progress: Higher temps, better error correction
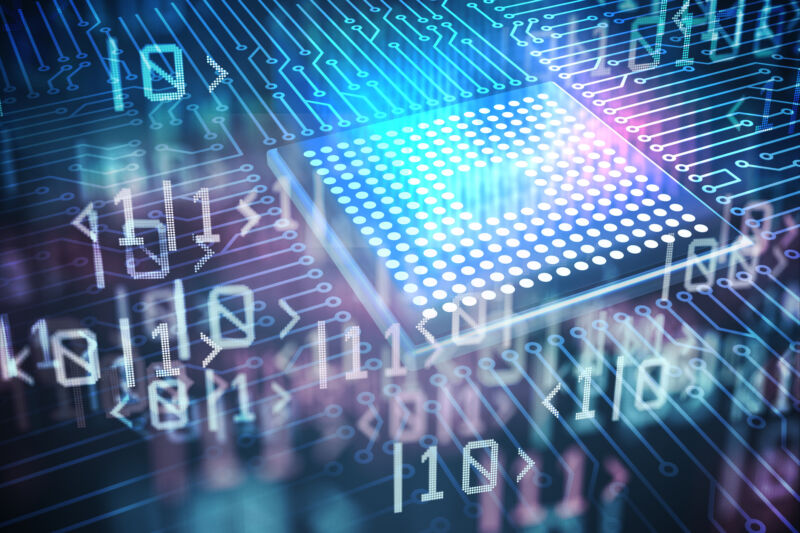
There’s a strong consensus that tackling most useful problems with a quantum computer will require that the computer be capable of error correction. There is absolutely no consensus, however, about what technology will allow us to get there. A large number of companies, including major players like Microsoft, Intel, Amazon, and IBM, have all committed to different technologies to get there, while a collection of startups are exploring an even wider range of potential solutions.
We probably won’t have a clearer picture of what’s likely to work for a few years. But there’s going to be lots of interesting research and development work between now and then, some of which may ultimately represent key milestones in the development of quantum computing. To give you a sense of that work, we’re going to look at three papers that were published within the last couple of weeks, each of which tackles a different aspect of quantum computing technology.
Hot stuff
Error correction will require connecting multiple hardware qubits to act as a single unit termed a logical qubit. This spreads a single bit of quantum information across multiple hardware qubits, making it more robust. Additional qubits are used to monitor the behavior of the ones holding the data and perform corrections as needed. Some error correction schemes require over a hundred hardware qubits for each logical qubit, meaning we’d need tens of thousands of hardware qubits before we could do anything practical.
A number of companies have looked at that problem and decided we already know how to create hardware on that scale—just look at any silicon chip. So, if we could etch useful qubits through the same processes we use to make current processors, then scaling wouldn’t be an issue. Typically, this has meant fabricating quantum dots on the surface of silicon chips and using these to store single electrons that can hold a qubit in their spin. The rest of the chip holds more traditional circuitry that performs the initiation, control, and readout of the qubit.
This creates a notable problem. Like many other qubit technologies, quantum dots need to be kept below one Kelvin in order to keep the environment from interfering with the qubit. And, as anyone who’s ever owned an x86-based laptop knows, all the other circuitry on the silicon generates heat. So, there’s the very real prospect that trying to control the qubits will raise the temperature to the point that the qubits can’t hold onto their state.
That might not be the problem that we thought, according to some work published in Wednesday’s Nature. A large international team that includes people from the startup Diraq have shown that a silicon quantum dot processor can work well at the relatively toasty temperature of 1 Kelvin, up from the usual milliKelvin that these processors normally operate at.
The work was done on a two-qubit prototype made with materials that were specifically chosen to improve noise tolerance; the experimental procedure was also optimized to limit errors. The team then performed normal operations starting at 0.1 K, and gradually ramped up the temperatures to 1.5 K, checking performance as they did so. They found that a major source of errors, state preparation and measurement (SPAM), didn’t change dramatically in this temperature range: “SPAM around 1 K is comparable to that at millikelvin temperatures and remains workable at least until 1.4 K.”
The error rates they did see depended on the state they were preparing. One particular state (both spin-up) had a fidelity of over 99 percent, while the rest were less constrained, at somewhere above 95 percent. States had a lifetime of over a millisecond, which qualifies as long-lived int he quantum world.
All of which is pretty good, and suggests that the chips can tolerate reasonable operating temperatures, meaning on-chip control circuitry can be used without causing problems. The error rates of the hardware qubits are still well above those that would be needed for error correction to work. However, the researchers suggest that they’ve identified error processes that can potentially be compensated for. They expect that the ability to do industrial-scale manufacturing will ultimately lead to working hardware.
Quantum computing progress: Higher temps, better error correction Read More »