AI trains on kids’ photos even when parents use strict privacy settings
“Outrageous” —
Even unlisted YouTube videos are used to train AI, watchdog warns.
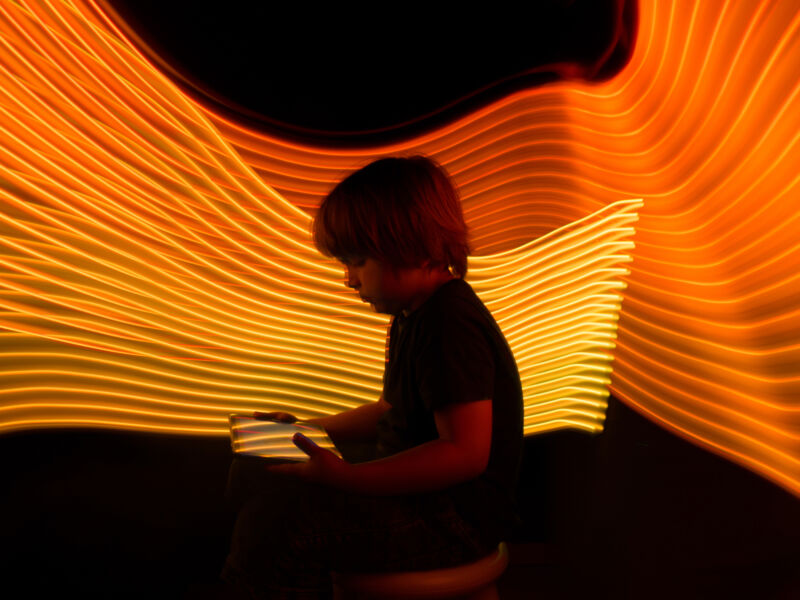
Human Rights Watch (HRW) continues to reveal how photos of real children casually posted online years ago are being used to train AI models powering image generators—even when platforms prohibit scraping and families use strict privacy settings.
Last month, HRW researcher Hye Jung Han found 170 photos of Brazilian kids that were linked in LAION-5B, a popular AI dataset built from Common Crawl snapshots of the public web. Now, she has released a second report, flagging 190 photos of children from all of Australia’s states and territories, including indigenous children who may be particularly vulnerable to harms.
These photos are linked in the dataset “without the knowledge or consent of the children or their families.” They span the entirety of childhood, making it possible for AI image generators to generate realistic deepfakes of real Australian children, Han’s report said. Perhaps even more concerning, the URLs in the dataset sometimes reveal identifying information about children, including their names and locations where photos were shot, making it easy to track down children whose images might not otherwise be discoverable online.
That puts children in danger of privacy and safety risks, Han said, and some parents thinking they’ve protected their kids’ privacy online may not realize that these risks exist.
From a single link to one photo that showed “two boys, ages 3 and 4, grinning from ear to ear as they hold paintbrushes in front of a colorful mural,” Han could trace “both children’s full names and ages, and the name of the preschool they attend in Perth, in Western Australia.” And perhaps most disturbingly, “information about these children does not appear to exist anywhere else on the Internet”—suggesting that families were particularly cautious in shielding these boys’ identities online.
Stricter privacy settings were used in another image that Han found linked in the dataset. The photo showed “a close-up of two boys making funny faces, captured from a video posted on YouTube of teenagers celebrating” during the week after their final exams, Han reported. Whoever posted that YouTube video adjusted privacy settings so that it would be “unlisted” and would not appear in searches.
Only someone with a link to the video was supposed to have access, but that didn’t stop Common Crawl from archiving the image, nor did YouTube policies prohibiting AI scraping or harvesting of identifying information.
Reached for comment, YouTube’s spokesperson, Jack Malon, told Ars that YouTube has “been clear that the unauthorized scraping of YouTube content is a violation of our Terms of Service, and we continue to take action against this type of abuse.” But Han worries that even if YouTube did join efforts to remove images of children from the dataset, the damage has been done, since AI tools have already trained on them. That’s why—even more than parents need tech companies to up their game blocking AI training—kids need regulators to intervene and stop training before it happens, Han’s report said.
Han’s report comes a month before Australia is expected to release a reformed draft of the country’s Privacy Act. Those reforms include a draft of Australia’s first child data protection law, known as the Children’s Online Privacy Code, but Han told Ars that even people involved in long-running discussions about reforms aren’t “actually sure how much the government is going to announce in August.”
“Children in Australia are waiting with bated breath to see if the government will adopt protections for them,” Han said, emphasizing in her report that “children should not have to live in fear that their photos might be stolen and weaponized against them.”
AI uniquely harms Australian kids
To hunt down the photos of Australian kids, Han “reviewed fewer than 0.0001 percent of the 5.85 billion images and captions contained in the data set.” Because her sample was so small, Han expects that her findings represent a significant undercount of how many children could be impacted by the AI scraping.
“It’s astonishing that out of a random sample size of about 5,000 photos, I immediately fell into 190 photos of Australian children,” Han told Ars. “You would expect that there would be more photos of cats than there are personal photos of children,” since LAION-5B is a “reflection of the entire Internet.”
LAION is working with HRW to remove links to all the images flagged, but cleaning up the dataset does not seem to be a fast process. Han told Ars that based on her most recent exchange with the German nonprofit, LAION had not yet removed links to photos of Brazilian kids that she reported a month ago.
LAION declined Ars’ request for comment.
In June, LAION’s spokesperson, Nathan Tyler, told Ars that, “as a nonprofit, volunteer organization,” LAION is committed to doing its part to help with the “larger and very concerning issue” of misuse of children’s data online. But removing links from the LAION-5B dataset does not remove the images online, Tyler noted, where they can still be referenced and used in other AI datasets, particularly those relying on Common Crawl. And Han pointed out that removing the links from the dataset doesn’t change AI models that have already trained on them.
“Current AI models cannot forget data they were trained on, even if the data was later removed from the training data set,” Han’s report said.
Kids whose images are used to train AI models are exposed to a variety of harms, Han reported, including a risk that image generators could more convincingly create harmful or explicit deepfakes. In Australia last month, “about 50 girls from Melbourne reported that photos from their social media profiles were taken and manipulated using AI to create sexually explicit deepfakes of them, which were then circulated online,” Han reported.
For First Nations children—”including those identified in captions as being from the Anangu, Arrernte, Pitjantjatjara, Pintupi, Tiwi, and Warlpiri peoples”—the inclusion of links to photos threatens unique harms. Because culturally, First Nations peoples “restrict the reproduction of photos of deceased people during periods of mourning,” Han said the AI training could perpetuate harms by making it harder to control when images are reproduced.
Once an AI model trains on the images, there are other obvious privacy risks, including a concern that AI models are “notorious for leaking private information,” Han said. Guardrails added to image generators do not always prevent these leaks, with some tools “repeatedly broken,” Han reported.
LAION recommends that, if troubled by the privacy risks, parents remove images of kids online as the most effective way to prevent abuse. But Han told Ars that’s “not just unrealistic, but frankly, outrageous.”
“The answer is not to call for children and parents to remove wonderful photos of kids online,” Han said. “The call should be [for] some sort of legal protections for these photos, so that kids don’t have to always wonder if their selfie is going to be abused.”
AI trains on kids’ photos even when parents use strict privacy settings Read More »